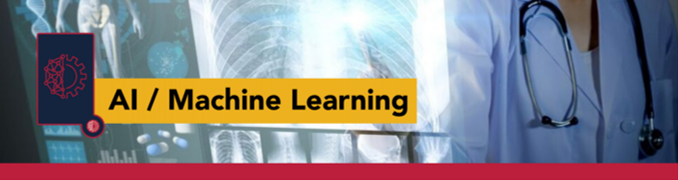
State of the Art
AI and machine learning is becoming an essential part of a broad range of application and system design, function and services, supporting increasingly naturalistic methods of communication, facial / gestural recognition, machine vision, autonomous robotics, complex data analytics, expert systems, and a broad range of reasoning strategies. In gaming and simulation, AI / machine learning can provide non-player characters (NPCs) with increasingly complex behaviour and, through player behavioural data analysis, adaptive strategies for support or competition.
Aspirations
AI / machine learning will be a critical component in creating intelligent, adaptive simulations, through three functions: 1) increasing the fidelity of the simulation through advanced NPC capabilities and interactivity; 2) data analytics and, the discovery of complex data patterns and predictive relationships; and, 3) adaptive system response based on simulation parameter (e.g. scenario scene complexity) modulation.
Challenges / barriers
The major barriers are associated with data analytics and pattern and predictive discovery. The granularity of the data being captured makes it truly “big data”, and data processing will be computationally intensive. Data modeling and mapping, and the selection of effective learning strategies will be key to discovering the correlational and predictive patterns between perceptual, cognitive, affective, behavioural and communication performance.
Immediate Research Projects: Enhancing simulation fidelity (e.g. increasing NPC speech interactivity).
- Developing data and stochastic models for identifying discrete data relationships (e.g. between pupillometry, gaze, visual event and heart rate data).
- Exploration and selection of data mining and machine learning frameworks to maximize predictive relationship discovery.
Longer Term Research Objectives:
- Develop a data capture infrastructure that can be utilized as a distributed environment to maximize data gathering.
- Expand and refine simulation state, data capture, analysis and AI / machine learning loop to maximize learner classification and response accuracy.
- Expand and refine simulation modulation strategies to enable highly adaptive, real time system response to learner level of expertise, cognitive and affective readiness.
Educational Validation
Simulation as a pedagogical strategy requires that it be appropriately scaled and focused to the level of the learner. AI can mediate a number of simulation parameters such as fidelity and scenario complexity. It can also, through continuous analysis and modification of simulation parameters and learner response, offer a platform through which the relationship between the simulation environment and learner perceptual, cognitive, affective, motoric and communication performance can be determined.