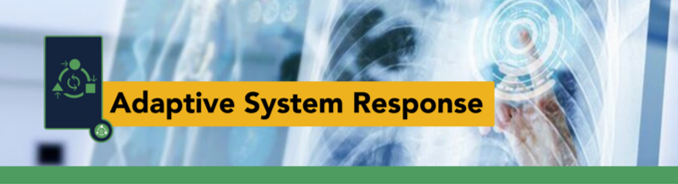
State of the Art
Simulation and gaming offer increasingly complex interplay between the player or learner and virtual environments. From AlphaGo, chess and first person shooter games, to the military’s adoption of “Live Synth” simulation training, the range of dynamic adaptation is growing to include enhanced non-player behavioural complexity and interactivity, and game machine learning. However, this adaptivity is not generally directly based on models of expertise (skills, competencies and benchmarks), learner development, learner levels of expertise and current learner state.
Aspirations
We believe that we can significantly improve simulation pedagogy by enhancing the understanding of the relationships between the simulation environment, learner levels of expertise, models of progression, and their perceptual, cognitive, affective, motoric and communication performance profiles. Identifying and automatically and dynamically responding to detected levels of learner expertise and cognitive state can significantly enhance learning outcomes, and provide a platform for new insights in assessment and performance.
Challenges / barriers
The ability to adaptively respond to a learner’s level of expertise and cognitive readiness is predicated on the following three challenges: 1) accurately identifying and classifying learner levels of expertise and current state based on performance data; 2) understanding the relationships between simulation parameters modulation (such as fidelity) and learning outcomes; and, 3) creating an infrastructure that can, based on models of learner progression and learner state, select and implement the most effective learning strategy for that learner
Immediate Research Projects: Developing a simulation environment that can be modulated across key areas such as fidelity, scenario and NPG
complexity, and temporal compression.
- Research relating simulation parameter modulation on learner performance and learning outcomes across levels of expertise.
Longer Term Research Objectives: Creating a non-domain specific, valid and reliable model which links simulation parameters, learner performance and learning outcomes.
- Develop a robust framework which enables the incorporation and modeling—for a specific domain—of the development of learner skills, competencies and expertise.
- Creating dynamic, real time simulation modulation and monitoring that continuously adjusts simulation parameters to match learner expertise, learner progression and current state
Educational Validation
Adaptive response to learner cognitive load, expertise and current state to support effective skills and competency development, in any domain, is the role of education. Great educators modulate learning activities to reflect, accommodate and support learners in a manner that extends, and does not disrupt, learner progress. Great educators also provide immediate and dynamic learner feedback that aids in that progress. We believe that we can emulate both facets in an intelligent, automated and dynamic simulation framework.