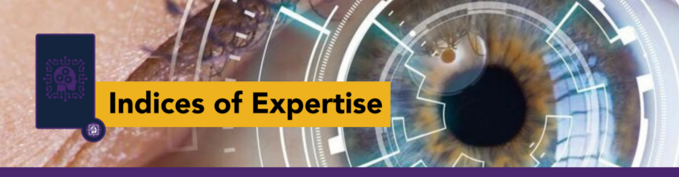
State of the Art
Expertise across domains is associated with several key attributes: breadth of procedural and declarative knowledge, available response repertoire, speed and quality of response execution, high perceptual and cognitive efficiency, enhanced situational awareness, communication efficacy, accurate outcome prediction, affect self-regulation, and enhanced self-efficacy. Despite their value as markers of expertise, assessing these attributes remains relatively blunt, cumbersome and resource intensive.
Aspirations By triangulating neurocognitive, physiological, behavioural and communication performance data, we believe that
we can:
- reliably identify indices of expertise that will provide objective evaluation and achievement of competencies.
- leverage the data to provide a real time adaptive, intelligent simulation response. Adaptive response parameters could include modulating scenario fidelity, complexity, temporal constraints and task difficulty. The result will be a learning experience that tailors the difficulty to optimize learning.
Challenges / barriers
While capturing the discrete data elements within each identified category of performance—perceptual, cognitive, affective, behavioural and motoric—does not represent a significant challenge, creating valid, reliable markers indicative of levels of expertise will require a sufficiently large data pool and sophisticated data modeling and analysis. Markers of more complex constructs such as situational awareness, strategic decision making, and effective leadership will require a combination of individual and group performance, and simulation scenario data.
Immediate Research Projects: Utilize the data capture infrastructure to gather data on expert and non-expert performance, both in situ and in
simulation environments;
- Compare performance metrics for all levels of expertise within both environments to determine correspondence in data expression.
- Develop initial data mining and analytics infrastructure and machine learning algorithms for classifying levels of expertise.
Longer Term Research Objectives:
- Develop a valid and reliable performance assessment toolkit that can be transferred across domains.
- Derive valid and reliable indices of expertise for both lower and higher level performance constructs.
- Create a model for identifying indices of expertise that are not domain specific.
- Maximize predictive efficiency through “minimized data, highest value”.
Educational Validation
Identifying valid and reliable indices of expertise is a central pillar supporting the ability to develop intelligent and adaptive simulation. While expertise is clearly indexed in all professional disciplines, methods of assessing learner expertise often remains opaque and poorly understood. We can, through highly granular data capture and intelligent data analysis, significantly contribute to creating meaningful and objective measures for learner assessment.